This post started with the post below on Facebook

I was curious if by chance some bacteria produced it. To find such information you must start with KEGG: Kyoto Encyclopedia of Genes and Genomes. On that site we find a description of the condition, we also find information about this enzyme glucose-6-phosphate dehydrogenase (NADP+) EC 1.1.1.49
Since it is deficiency, we look for an alternative supply from bacteria in the microbiome. There are many bacteria that has the capacity of producing it, but the enzyme may not be turned on (epigenetics). I was directed by perplexity to Wikipedia. This identifies a bacteria that is likely a high (actual) producer.
Leuconostoc mesenteroides: This bacterium has been shown to possess a G6PD enzyme that is reactive toward 4-hydroxynonenal, in addition to glucose-6-phosphate
This bacteria is available as a probiotic (one source).
General Information about Autism Enzymes and Compound Production
From the hundreds of donated microbiome samples annotated with Autism on Microbiome Prescription, I have done some statistical analysis (using a patent pending method for partitioning samples), “poor man metagenomics”, and have produce a summary on those who have an official diagnosis of autism.
First, I checked if EC 1.1.1.49 was on the list. It was not, which implies that is not a very common item across all autism patients.
Browsing the lists I did find two familiar items being high:
- (R)-Lactate – also known as d-lactic acid, a common cause of brain fog and other neurological conditions see this for a list.
- L-Histidine which is likely a protective feature (more information)
The amount of (R)-Lactate reported above was computed from the microbiome data which implies that reducing its level by microbiome manipulation is a viable path.
Modelling on an individual sample
With recent revisions of the UI, I have built an algorithm to select the probiotics that supply the maximum amount of these KEGG compounds and Enzymes that most meet the deficiencies detected.
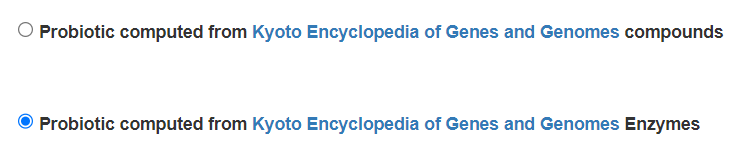
To illustrate this feature, I took one of the autism samples upload and ask for the suggestions.
Below is the results of probiotics to increase the low KEGG compound in this sample.
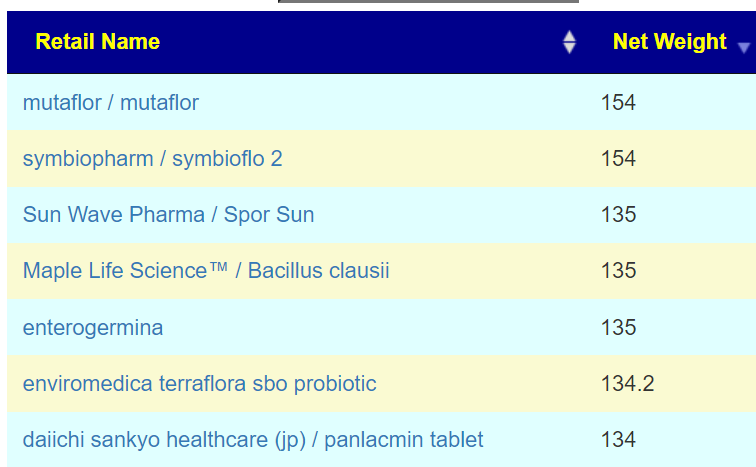
Below is the results of probiotics to increase the low KEGG Enzymes in this sample. They are reasonably close to each other.
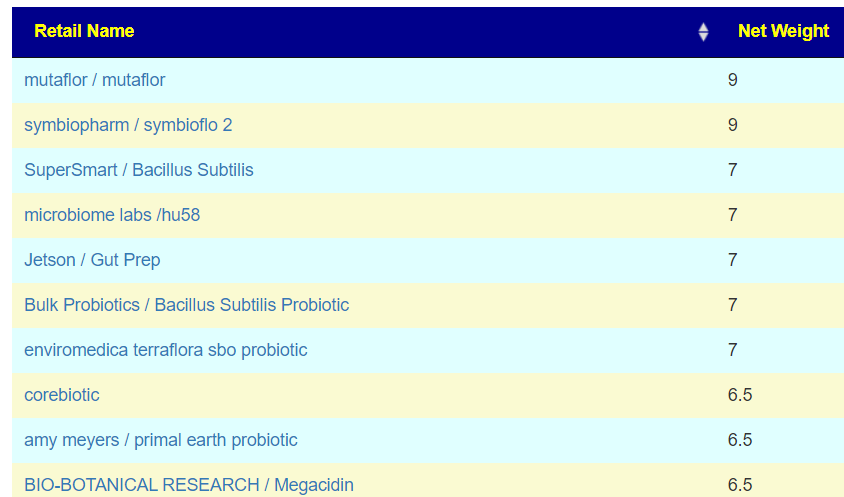
Bottom Line
This is all theoretical, but as probiotics are usually deemed to be safe and without significant risks, it is a possible experiment to try. Always take detail notes and report benefits and problems as comments on this post
It is interesting to note that the common Lactobacillus and Bifidobacterium are not need the top of either list.
An older video on the process
Postscript and Reminder
As a statistician with relevant degrees and professional memberships, I present data and statistical models for evaluation by medical professionals. I am not a licensed medical practitioner and must adhere to strict laws regarding the appearance of practicing medicine. My work focuses on academic models and scientific language, particularly statistics. I cannot provide direct medical advice or tell individuals what to take or avoid.My analyses aim to inform about items that statistically show better odds of improving the microbiome. All suggestions should be reviewed by a qualified medical professional before implementation. The information provided describes my logic and thinking and is not intended as personal medical advice. Always consult with your knowledgeable healthcare provider.
Implementation Strategies
- Rotate bacteria inhibitors (antibiotics, herbs, probiotics) every 1-2 weeks
- Some herbs/spices are compatible with probiotics (e.g., Wormwood with Bifidobacteria)
- Verify dosages against reliable sources or research studies, not commercial product labels. This Dosages page may help.
- There are 3 suppliers of probiotics that I prefer: Custom Probiotics , Maple Life Science™, Bulk Probiotics: see Probiotics post for why
- My preferred provider for herbs etc is Maple Life Science™ – they are all organic, fresh, without fillers, and very reasonably priced.
Professional Medical Review Recommended
Individual health conditions may make some suggestions inappropriate. Mind Mood Microbes outlines some of what her consultation service considers:
A comprehensive medical assessment should consider:
- Terrain-related data
- Signs of low stomach acid, pancreatic function, bile production, etc.
- Detailed health history
- Specific symptom characteristics (e.g., type and location of bloating)
- Potential underlying conditions (e.g., H-pylori, carbohydrate digestion issues)
- Individual susceptibility to specific probiotics
- Nature of symptoms (e.g., headache type – pressure, cluster, or migraine)
- Possible histamine issues
- Colon acidity levels
- SCFA production and acidification needs
A knowledgeable medical professional can help tailor recommendations to your specific health needs and conditions.
Recent Comments